Laurent Lefèvre participe à l’organisation de la conférence « Ecrans : menaces sur la santé ».
Celle-ci se déroulera à l’Amphithéâtre Descartes, Site Descartes, Ecole Normale Supérieure de Lyon de Lyon le 9 Mai 2023.
Non classé
Phd Ghoshana Bista: Total cost modeling of software ownership in Virtual Network Functions
– English version below –
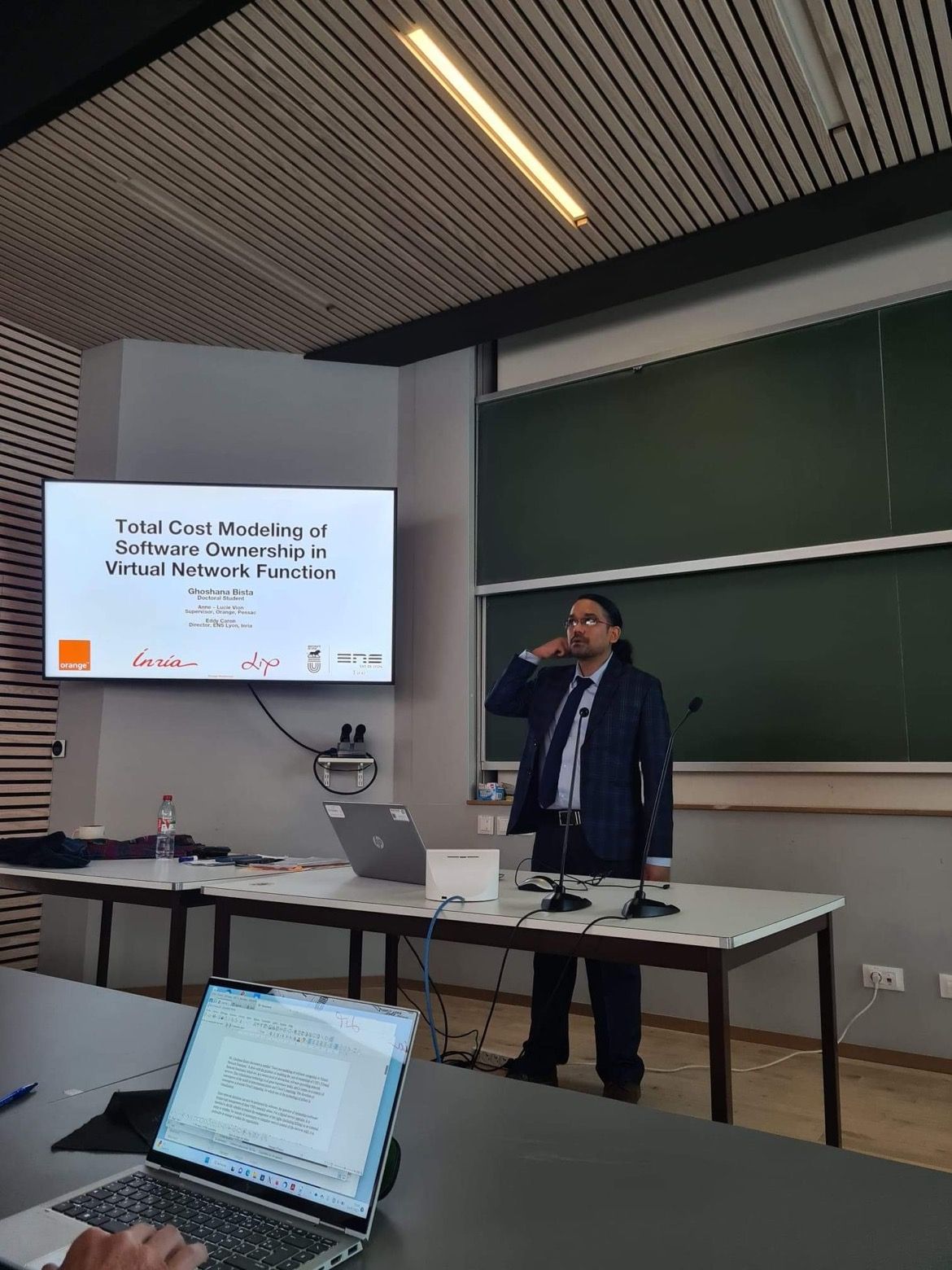
J’ai le plaisir de vous inviter à ma soutenance de thèse intitulée :
« Modélisation du coût total de la propriété du logiciel dans Virtual Network Functions»
Cette soutenance (en Anglais) le mardi 24 janvier à 10 h :
Lieu : ENS LYON LABORATOIRE LIP 46 ALLEE D’ITALIE 69007, LYON, France, Salle des Thèse (Rdc)
Devant le jury composé de :
- Rapporteur Christophe Cérin Professeur des Universités Université Sorbonne Paris Nord
- Rapporteur Jean-Frederic Myoupo Professeur des Universités Université de Picardie Jules Verne
- Examinateur Franck Petit Professeur des Universités Université Pierre et Marie Curie Paris 6
- Examinatrice Joanna Moulierac Maître de Conférences Université Côte d’Azur
- Examinateur Noel De Palma Professeur des universités Université Grenoble Alpes
- Directeur Eddy Caron Maître de Conférences HDR ENS de Lyon
- Co-encadrante Anne-Lucie Vion Responsable du groupe SAM Orange
Résumé :
Aujourd’hui, un changement massif est en cours dans les réseaux de télécommunication avec l’émergence de la softwarisation et de la cloudification. Parmi les technologies qui accompagnent ces mutations, l’une d’elles est la NFV (Network Function Virtualization). NFV est l’architecture réseau qui découple les fonctions réseau des périphériques matériels (middleboxes) à l’aide d’un composant virtuel appelé VNF (Virtual Network Function). VNF a changé le paradigme technologique des réseaux. Avant : la fonction de réseau était assurée par un équipement physique et les fournisseurs de services acquéraient sa propriété pour la durée de vie du matériel de confiance (au lieu de compter en années). Aujourd’hui, les fonctions réseau sont des logiciels que les fournisseurs de services développent ou acquièrent en achetant des licences. Une licence définit le droit d’utilisation (RTU) du logiciel.
Par conséquent, si l’octroi de licences dans la NFV n’est pas géré de manière appropriée, les fournisseurs de services pourraient (1) être exposés à la contrefaçon et risquer de lourdes sanctions financières en cas de non-conformité ; (2) pourrait suracheter des licences pour couvrir des usages mal estimés. Ainsi, la maîtrise de la licence de fonction réseau via la mise en place du Software Asset Management et du FinOps (Finance et DevOps) est indispensable pour maîtriser les coûts. Dans cette recherche, notre problème principal est de minimiser le TCO (Total Cost of Ownership) du coût du logiciel (VNF), en fournissant une Qualité de Services (QoS) à un nombre spécifique d’utilisateurs. Les coûts des logiciels comprennent divers coûts, du développement à la maintenance, de l’intégration à la gestion des versions et des services professionnels. Nos recherches portent sur les logiciels propriétaires (développés par un éditeur et vendus via une licence payante). Nous avons considéré que le TCO se compose du coût de la licence du logiciel, des ressources nécessaires pour exécuter et faire fonctionner le SW, et de l’énergie consommée par cette exécution. Dans cette recherche, premièrement, nous avons identifié le besoin d’un modèle de licence VNF standardisé, qui dépend fortement de la créativité du fournisseur VNF ; Cette absence de normes expose les CSP (Communication Service Providers) au risque de devoir déléguer la gestion des droits aux fournisseurs. Par conséquent, nous avons proposé un modèle de licence basé sur les métriques, qui aide à quantifier l’utilisation du VNF. Après avoir estimé la licence de VNF, nous avons estimé le coût de la licence. Ensuite, nous avons présenté plusieurs façons de minimiser le coût de la licence en fonction des différents cas d’utilisation, qui dépendent du scénario et des besoins de l’utilisateur. Puis après, avec l’aide des connaissances industrielles, nous avons constaté que la réduction de la consommation de ressources pour minimiser le TCO fournissant la QoS affecte le déploiement de la VNF directement ou indirectement, ce qui impacte l’octroi de licences. Ainsi, les licences et les ressources sont interdépendantes. Nous avons utilisé ces coûts pour construire le coût total du logiciel. Après cela, nous avons proposé plusieurs façons de réduire le coût total du logiciel en répondant aux exigences du client. Puis après, nous avons considéré l’énergie et son coût associé de VNF. La consommation énergétique du VNF est dépendante de la consommation des ressources, et les usages des ressources impactent la licence. Ainsi, on voit que ces trois coûts sont interdépendants : licence, ressources et coût énergétique de VNF. Par conséquent, nous considérons ces coûts et le TCO construit. Minimiser le coût total de possession en répondant aux exigences du client est un défi car il s’agit d’un multi-paramètres. Par conséquent, nous avons proposé plusieurs algorithmes heuristiques basés sur le partage et la consolidation des ressources pour réduire le TCO en fonction de la licence, de la préférence des ressources et des scénarios du client.
I am pleased to invite you to my thesis defense entitled:
« Total cost modeling of software ownership in Virtual Network Functions»
This defense (in English) will be on Tuesday, January 24th, at 10 am
At : ENS LYON LABORATOIRE LIP 46 ALLEE D’ITALIE 69007, LYON, France
The jury will be composed of the following persons:
- Reviewer Christophe Cérin Professor des Universités Université Sorbonne Paris Nord
- Reviewer Jean-Frederic Myoupo Professor des Universités Université de Picardie Jules Verne
- Examiner Franck Petit Professor des Universités Université Pierre et Marie Curie Paris 6
- Examiner Joanna Moulierac Maître de Conférences Université Côte d’Azur
- Examiner Noel De Palma Professor des universités Université Grenoble Alpes
- Director Eddy Caron Maître de Conférences HDR ENS de Lyon
- Superviser Anne-Lucie Vion Responsable du groupe SAM Orange
Abstract :
Today, a massive shift is ongoing in telecommunication networks with the emergence of softwarization and cloudification. Among the technologies which are assisting these shifts, one of them is NFV (Network Function Virtualization). NFV is the network architecture that decouples network functions from hardware devices (middleboxes) with the help of a virtual component known as VNF (Virtual Network Function). VNF has shifted the network technological paradigm. Before: Network Function was performed by physical equipment, and service providers acquired its property for the lifetime of the relying hardware (instead counted in years). Today, Network functions are software that service providers develop or acquire purchasing licenses. A license defines software’s Right to Use (RTU).
Therefore, if licensing in NFV is not appropriately managed, service providers might (1) be exposed to counterfeiting and risk heavy financial penalties due to non-compliance; (2) might overbuy licenses to cover poorly estimated usages. Thus, mastering network function license through implementing Software Asset Management and FinOps (Finance and DevOps) is essential to control costs. In this research, our primary problem is to minimize the TCO (Total Cost of Ownership) of software cost (VNF), providing Quality of Services (QoS) to a specific amount of users. Software costs include various costs, from development to maintenance, integration to release management, and professional services. Our research focuses on proprietary software (developed by a publisher and sold via a paid license).
We considered that TCO consists of the software license cost, the resources necessary to execute and operate SW, and the energy consumed by this execution. In this research, first, we have identified the need for a standardized VNF licensing model, which is highly dependent on the VNF provider’s creativity; This lack of standards places CSPs (Communication Service Providers) at risk of having to delegate the management of rights to suppliers. Hence, we proposed a licensing model based on the metrics, which help to quantify the usage of the VNF. After estimating the license of VNF, we estimated the license cost. Afterward, we presented several ways to minimize the license cost depending upon the different use cases, which depend on the user’s scenario and needs. Then after, with the help of industrial knowledge, we found that reducing resource consumption to minimize the TCO providing QoS affects the deployment of the VNF directly or indirectly, which impacts the licensing. Thus, the licenses and resources are interdependent. We used these costs to construct the software’s total cost. After that, we proposed several ways to reduce the software’s total cost by fulfilling the client’s requirements. Then after, we considered the energy and its associated cost of VNF. The energy consumption of the VNF is dependent on resource consumption, and resources usages impact the license. Thus, we can see that these three costs are interdependent: license, resources, and energy cost of VNF. Hence, we consider these costs and constructed TCO. Minimizing TCO fulfilling the client’s requirements is challenging since it is a multi-parameter. Therefore, we proposed several heuristical algorithms based on resource sharing and consolidation to reduce the TCO depending on the license, resource preference, and the client’s scenarios.
Avalon à la Fête de la Science 2020
Laurent Lefevre has participated to the “Fête de la Science 2020” (Science Festival) for Inria on the topic “Pollution Numérique, tous responsables ?” – “Digital Pollution, all responsibles ?”, October 6, 2020.
The video (in French) can viewed here : https://www.youtube.com/watch?v=Wwqy7g2m9P0&feature=youtu.be
The 2019 edition of the Avalon activity report is available!
The Avalon 2019 report is available as well as all activity reports since 2012.
Avalon is contributing to LLVM OpenMP/runtime
Philippe Virouleau, funding thanks to EoCoE-II project, has proposed patches to LLVM OpenMP/runtime in order to provide better control and performances of OpenMP task execution. First accepted patch was pushed in LLVM master branch (https://reviews.llvm.org/D63196). It solves an side effect due to the task throttling heuristic that serializes task execution. It may cripple the application performance in some specific task graph scenarios, like the ones detailed in section 4.2 from this paper published at IWOMP 2018 (the full text can be found here). In such cases not having the full task graph prevent some opportunities for cache reuse between successive tasks.
Mid term goals are to transfer some important and innovative features mostly already available in libKOMP https://gitlab.inria.fr/openmp/libkomp.
WG – Alba Cristina Magalhaes Alves de Melo: Parallel Sequence Alignment of Whole Chromosomes with Hundreds of GPUs and Pruning
2018-02-28
Title: Parallel Sequence Alignment of Whole Chromosomes with Hundreds of
GPUs and Pruning
Speaker: Alba Cristina Magalhaes Alves de Melo
Abstract: Biological Sequence Alignment is a very basic operation in Bioinformatics used routinely worldwide. Smith-Waterman is the exact algorithm used to compare two sequences, obtaining the optimal alignment in quadratic time and space. In order to accelerate Smith-Waterman, many GPU-based strategies were proposed in the literature. However, aligning DNA sequences of millions of characters, or Base Pairs (MBP), is still a very challenging task. In this talk, we discuss related work in the area of parallel biological sequence alignment and present our multi-GPU strategy to align DNA sequences with up to 249 millions of characters in 384 GPUs. In order to achieve this, we propose an innovative speculation technique, which is able to parallelize a phase of the Smith-Waterman algorithm that is inherently sequential. We combined our speculation technique with sophisticated buffer management and fine-grain linear space matrix processing strategies to obtain our parallel algorithm. As far as we know, this is the first implementation of Smith-Waterman able to retrieve the optimal alignment between sequences with more than 50
millions of characters. We will also present a pruning technique for one GPU that is able to prune more than 50% of the Smith-Waterman matrix and still retrieve the optimal alignment. We will show the results obtained in the Keeneland cluster (USA), where we compared all the human x chimpanzee homologous chromosomes (ranging from 26 MBP to 249 MBP). The human_chimpanzee chromosome 5 comparison (180 MBP x 183 MBP) attained 10.35 TCUPS (Trillions of Cells Updated per Second) using 384 GPUs. In this case, we processed 45 petacells, being able to produce the optimal alignment in 53 minutes and 7 seconds, with a speculation hit ratio of 98.2%.
Speaker Bio: Alba Cristina Magalhaes Alves de Melo obtained her PhD degree in Computer Science from the Institut National Polytechnique de Grenoble (INPG), France, in 1996. In 2008, she did a postdoc at the University of Ottawa, Canada; in 2011, she was invited as Guest Scientist at Université Paris-Sud, France; and in 2013 she did a sabbatical at the Universitat Polytecnica de Catalunya, Spain. Since 1997, she works at the Department of Computer Science at the University of Brasilia (UnB), Brazil, where she is now a Full Professor. She is also a CNPq Research Fellow level 1D in Brazil. She was the Coordinator of the Graduate Program in Informatics at UnB for several years (2000-2002, 2004-2006, 2008, 2010, 2014) and she coordinated international collaboration projects with the Universitat Politecnica de Catalunya, Spain (2012, 2014-2016) and with the University of Ottawa, Canada (2012-2015). In 2016, she received the Brazilian Capes Award on “Advisor of the Best PhD Thesis in Computer Science”. Her research interests are High Performance Computing, Bioinformatics and Cloud Computing. She advised 2 postdocs, 4 PhD Thesis and 22 MsC Dissertations. Currently, she advises 4 PhD students and 2 MsC students. She is Senior Member of the IEEE Society and Member of the Brazilian Computer Society. She gave invited talks at Universitat Karlshure, Germany, Université Paris-Sud, France, Universitat Polytecnica de Catalunya, Spain, University of Ottawa, Canada and at Universidad del Chile, Chile. She has currently 91 papers
listed at DBLP (www.informatik.uni-trier.de/~ley/db/indices/a-tree/m/Melo:Alba_Cristina_Magalhaes_Alves_de.html).
DIET goes fishing at Rutgers University
The Workflow DIET engine plays with the Fishes Detection Application
In the context of SUSTAM (Associated joint team between Avalon and RDI2 Lab. in Rutgers University). Daniel Balouek-Thomert (RDI2), Eddy Caron (Avalon), Hadrien Croubois (Avalon) and Alireza Zamani (RDI2), worked on deployment of an application from the Ocean Observatories Initiative (OOI) project on Grid’5000 using the DIET Middleware.
Moreover, on Friday december 1st, Eddy and Hadrien give a talk about DIET and elastic workflow.
Abstract: Cloud platforms have emerged as a leading solution for accessing computational resources for everybody. However, high performance scientific computing is still among the few domains where Cloud still raises more questions than it solves. While it is today possible to use existing approaches to deploy scientific workflows on virtualized platforms, these approaches do not benefit of many new features offered by Cloud providers. Among these features is the possibility of having an elastic platform that reacts to the need of the users, therefore offering an improved Quality of Service (QoS) while reducing the deployment cost. In this talk, we will present the DIET toolbox, a middleware designed for high-performance computing in a heterogeneous and distributed environment and our recent work on elastic workflows.
![]() |
![]() |
![]() |
![]() |
Energy Efficient Traffic Engineering in Software Defined Networks [Radu Carpa. Thèse]
Soutenance de Thèse de Radu Carpa
Jeudi 26 Octobre. Amphi B. 14h00
Résumé
Ce travail a pour but d’améliorer l’efficacité énergétique des réseaux en éteignant un sous-ensemble de liens par une approche SDN. Nous nous différencions des nombreux travaux de ce domaine par une réactivité accrue aux variations des conditions réseaux. Cela a été rendu possible grâce à une complexité calculatoire réduite et une attention particulière au surcoût induit par les échanges de données.
L’architecture logicielle “SegmenT Routing based Energy Efficient Traffic Engineering” (STREETE) que nous proposons repose sur un re-routage dynamique du trafic en fonction de la charge du réseau. Grace à des méthodes d’équilibrage de charge, nous obtenons un placement presque optimal des flux dans le réseau.
STREETE a été validé sur une vraie plateforme SDN. Cela nous a permis de donner des indications sur des améliorations à prendre en compte afin d’éviter des instabilités causées par des basculements incontrôlés des flux réseau entre des chemins alternatifs.
Membres du Jury
- Andrzej DUDA, Professeur – Grenoble INP-Ensimag (Examinateur)
- Frédéric GIROIRE, Chargé de Recherches – CNRS Sophia Antipolis (Examinateur)
- Brigitte JAUMARD, Professeure – Concordia University, Canada (Rapporteure)
- Béatrice PAILLASSA, Professeure – Institut national polytechnique de Toulouse (Rapporteure)
- Laurent LEFEVRE, Chargé de Recherches – Inria ENS Lyon (Directeur)
- Olivier GLUCK, Maître de Conférences – UCBL Lyon1 (Co-encadrant)
ANR MapReduce
This project is devoted to using MapReduce programming paradigm on clouds and hybrid infrastructures. Partners: Argonne National Lab (USA), the University of Illinois at Urbana Champaign (USA), the UIUC-INRIA Joint Lab on Petascale Computing, IBM France, IBCP, MEDIT (SME) and the GRAAL/AVALON INRIA project-team.
This project aims to overcome the limitations of current Map-Reduce frameworks such as Hadoop, thereby enabling highly-scalable Map-Reduce-based data processing on various physical platforms such as clouds, desktop grids, or on hybrid infrastructures built by combining these two types of infrastructures.To meet this global goal, several critical aspects will be investigated. Data storage and sharing architecture. First, we will explore advanced techniques for scalable, high-throughput, concurrency-optimized data and metadata management, based on recent preliminary contributions of the partners. Scheduling. Second, we will investigate various scheduling issues related to large executions of Map-Reduce instances. In particular, we will study how the scheduler of the Hadoop implementation of Map-Reduce can scale over heterogeneous platforms; other issues include dynamic data replication and fair scheduling of multiple parallel jobs. Fault tolerance and security. Finally, we intend to explore techniques to improve the execution of Map-Reduce applications on large-scale infrastructures with respect to fault tolerance and security.
Our global goal is to explore how combining these techniques can improve the behavior of Map-Reduce-based applications on the target large-scale infrastructures. To this purpose, we will rely on recent preliminary contributions of the partners associated in this project, illustrated though the following main building blocks. BlobSeer, a new approach to distributed data management being designed by the KerData team from INRIA Rennes – Bretagne Atlantique to enable scalable, efficient, fine-grain access to massive, distributed data under heavy concurrency. BitDew, a data-sharing platform being currently designed by the GRAAL team from INRIA Grenoble – Rhône-Alpes at ENS Lyon, with the goal of exploring the specificities of desktop grid infrastructures. Nimbus, a reference open source cloud management toolkit developed at the University of Chicago and Argonne National Laboratory (USA) with the goal of facilitating the operation of clusters as Infrastructure-as-a-Service (IaaS) clouds.
More information on the MapReduce web site.
ANR COOP
Multi-level Cooperative Resource Management
The problem addressed by the COOP project (Dec. 2009 — May 2013) was to reconcile two layers – Programming Model Frameworks (PMF) and Resource Management Systems (RMS) – with respect to a number of tasks that they both try to handle independently. PMF needs to have a knowledge of resources to select the most efficient transformation of abstract programming concepts into executable ones. However, the actual management of resources is done by RMS in an opaque way, based on a simple abstraction of applications.
More details are available on the ANR COOP website.